All published articles of this journal are available on ScienceDirect.
Covalent Docking for Novel Series of Spiro-butenolides on Trx R1 for QSAR Modelling
Abstract
Background
The biological activity of the new series of 1'H,5H-spiro[furan-2,4'-isoquinoline]-1',3',5(2'H)-triones and their selectivity for selenocysteine inspired us to create a QSAR model for design new thioredoxin reductase inhibitors.
Results
The article provides an example of an analysis of the dependence of molecular descriptors and their relationship with the results of covalent docking for thioredoxin reductase. The constructed model makes it possible to predict the activity and selectivity of new electron-deficient olefins towards thioredoxin reductase.
Conclusion
A small set of molecular descriptors of shape, charges on carbon atoms and energy values of molecular orbitals makes it possible to quickly calculate the concentration of half-inhibition of thioredoxin reductase and allows the selection of molecules for subsequent synthesis.
1. INTRODUCTION
Thioredoxin reductase I (Trx R1) is an enzyme of the redox homeostasis system [1, 2]. In the last two decades, medicinal chemists and pharmacologists have shown great interest in inhibitors of this enzyme as therapeutic agents [3-7]. A number of Trx R1 inhibitors act by the mechanism of covalently binding to the selenocysteine residue at position 498, A, or C of the chain [8]. A common marker of Trx R1 inhibitory activity against organic compounds is the presence of a chemotype represented by electron-deficient olefins (Michael acceptors) [9-11]. Previously, Trx R1 inhibitors containing an electron-deficient olefin group, namely peptidomimetics based on (E)-4-(4-fluorophenyl)-4-oxobut-2-enoic acid were presented in a study [12]. The development and search for new compounds, the mechanism of action of which is based on the inhibition of Trx R1, requires solving the problem of selective action on the selenocysteine residue, since in living organisms cysteine residues and free SH-groups are present in proteins in large amounts [13]. The selectivity of Michael acceptors is analyzed using model systems based on cysteine and selenocysteine [14]. The obtained data on inhibitory activity against Trx R1 [15] led us to think about the possibility of constructing a QSAR model of covalent binding of inhibitors to Sec498 Trx R1. In our study, we used Trx R1 as a drug target because this newly anti-coogenic target for antimetastasis therapy and very important strategy in medicinal chemistry. The aim of this study is to conduct virtual screening based on compu- tational chemistry algorithms for the targeted search for novel thioredoxin reductase inhibitors, as similar methods have been successfully applied previously [16].
2. MATERIALS AND METHODS
2.1. Molecular Docking
2.1.1. Protein Preparation
The crystal structure of human TrxR1 was downloaded from RCSB PDB (Protein Data Bank) database (https://www.rcsb.org/) with PDBID 3EAN [17]. All ligands, ions and water molecules were removed from the structure. All hydrogen atoms were added to the protein structure and then truncated to only polar hydrogen atoms during the preparation process. Protein Preparation Wizard of Schrödinger Software Suite (Schrödinger, LLC: New York, NY, 2017) [18, 19]. During the process of noncovalent molecular docking, Sec498 on chain C of TXNRD dimer was mutated to alanine mimicking the first step of covalent docking, and the mass center of alanine was defined as the center of the binding site. A grid box with dimensions 20 × 20 × 20 and grid spacing 0.375 Å was placed in the center of the selenocysteine catalytic site of the protein receptor. Molecular docking was carried out in the Schrödinger Software Suite (Schrödinger, LLC: New York, NY, 2017). Covalent docking was performed around the reactive residue Cys497 and Cys498 within 25 Å. In LH-Trx1 covalent docking, the preparation of the protein Trx1. In Michael's addition script in the OPLS-2005 force field. The Sec498 on chain C was picked as the reactive residue for Michael's addition. During the pose prediction process, the cutoff of energy to retain poses was set as 2.5 kcal/mol and a maximum of 200 poses were retained for further refinement. The binding poses with the best docking score were picked out for the binding mode analysis. Binding energy calculated by MM-BGSA algorithm.
2.2. Ligand Preparation
The library of compounds with α, β-unsaturated carbonyl was prepared in the LigPrep module by adding a force field of MMFFs and generating possible states at a pH of 7.0 ± 2.0. Then, all the prepared compounds were docked using the Standard Precision (SP) docking protocol.4.4.3. Library of compounds (Supplementary material).
2.3. Docking on Trx R1
A grid box with dimensions 20 × 20 × 20 and grid spacing 0.375 Å was placed in the center of the selenocysteine catalytic site of the protein receptor. Molecular docking was carried out in the Schrödinger Software Suite (Schrödinger, LLC: New York, NY, 2017).
2.4. Covalent Docking on Trx R1
Covalent docking was performed around the reactive residue Cys497 and Cys498 within 25 Å. In LH-Trx1 covalent docking, the preparation of the protein Trx1. In Michael's addition script in the OPLS-2005 force field. The Sec 498 on chain C was picked as the reactive residue for Michael's addition. During the pose prediction process, the cutoff of energy to retain poses was set as 2.5 kcal/mol and a maximum of 200 poses were retained for further refinement. The binding poses with the best docking score were picked out for the binding mode analysis. Binding energy calculated by MM-BGSA algorithm.
2.5. Molecular Descriptor Calculation
Quantum chemical descriptors were calculated using the Sparatan 14 software package using the density functional algorithm (DFT) in B3lyp 6-31G basis set while taking into account water solvation.
2.6. QSAR (Quantity Structure-activity Relationship) and PLS (Partial Least Squares Regression)
A multiparameter model was built using the PLS method in the Graph Pad Prism software package for the most active and selective cases of Trx R1 inhibition. The relationship between the structure of these compounds and their biological activity was assessed using computational chemistry approaches. The calculation algorithm and its results are presented in ESI. We selected descriptors with a wide dynamic range. We found a significant contribution to the mathematical model of the dependence of thioredoxin reductase inhibition from the following quantities: the Muliken charge on the beta carbon atom of the spiral cyclic fragment of butenolide, the difference between the electrostatic charges on the beta and alpha atoms (Table 1). The potential energy of the enzyme-inhibitor complex is influenced by the descriptors of ovality, dipole moment, HOMO and polarizability.
2.7. Pharmacophore Modelling
The pharmacophore model of the spatial arrangement of substituents at the spiro-furanone fragment was modeled using the “Develop Pharmacophore Hypothesis tool” in the Schrodinger software package [20]. The descriptors we selected were used to construct a multiparameter model to predict the activity of covalent inhibitors of thioredoxin reductase I carrying in their structure a fragment of an electron-deficient olefin.
2.8. Colorimetric Detection of Cell Lysate TrxR Activity
For the TrxR assay, lysate from SH-SY5Y cells was used. A-548 cells were washed twice with phosphate buffer solution (PBS) and harvested from a flask with a scraper. Cells were homogenized in ice-cold 50 mM potassium phosphate buffer (pH 7.4), containing 1 mM EDTA, with Ultra Turrax T25 (Ikawerk, Janke and Kunkel Inc., Staufen, Germany). The homogenates were centrifuged at 10.000xg for 15 min at 4 °C. The protein concentrations of the supernatants were determined using a Bio-Rad protein assay kit (Bio-Rad Laboratories, USA). TrxR activity was assayed using 5,5- dithio-bis-(2-nitrobenzoic acid) (DTNB, Sigma-Aldrich, USA) as the substrate [12]. The reactions were run on 96 well plates, the final volume of 200 µl, in 50 mM potassium phosphate buffer (pH 7.4), containing 50 µg of the cell lysate proteins, 1mMEDTA, 50mMKCl, 0.2 mg/mL bovine serum albumin, 0.25 mM NADPH (Acros Organics, USA). The reaction mixture was incubated in 190 µl of reaction volume for 15 min on a plate shaker at room temperature, with compounds tested at appropriate concentrations. After incubation 10 µl of DTNB was added to a final concentration of 0.5 mM. Enzyme kinetics was monitored on the Clariostar microplate reader, by measuring the increase in absorbance at 412 nm for 20 min. The background TrxR-independent reduction of DTNB in the cell lysates was subtracted from each value. Aurum- tiomalate is used to validate inhibitor TRX.
S.No | Formula | IC50 µM |
---|---|---|
1 |
![]() |
5.90 ± 0.69 |
2 |
![]() |
1.49 ± 0.08 |
3 |
![]() |
3.86 ± 0.25 |
4 |
![]() |
4.92 ± 0.66 |
5 |
![]() |
17.64 ± 0.74 |
6 |
![]() |
53.71 ± 10.00 |
7 |
![]() |
40.06 ± 6.00 |
8 |
![]() |
8.87 ± 1.06 |
9 |
![]() |
171.70 ± 14.00 |
10 |
![]() |
117.64 ± 7.60 |
11 |
![]() |
17.78 ± 1.54 |
12 |
![]() |
26.07 ±3.04 |
13 |
![]() |
37.15 ± 3.64 |
14 |
![]() |
40.39± 2.35 |
15 |
![]() |
40.45± 5.52 |
16 |
![]() |
60.44 ± 6.00 |
17 |
![]() |
40.45± 5.52 |
18 |
![]() |
60.44 ± 6.00 |
19 |
![]() |
6.76 ± 0.65 |
20 |
![]() |
149.03 ±9.00 |
2.9. IC50 Calculation and Statistical Determination
For IC50 GraphPad Prism software and nonlinear regression for 3 times kinetic experiments, All graphs are present in the supplementary information. In Table 1, we present results for IC50.
3. RESULTS AND DISCUSSION
In our recent work on the inhibition activity of human thioredoxin reductase I by a new series of spirocyclic compounds, obtained using an innovative diazo-chemistry approach, selective inhibitors with high activity were discovered that selectively bind the selenol group of selenocysteine [21]. Measured IC50 value for training set present in tables supplementary information.
The structures of the Trx R1 inhibitors used for modeling and their half-inhibition concentration values. Calculation of the Gibson binding energy of the enzyme and inhibitor allows one to calculate the stability constant of the complex. This value strongly depends on the HOMO energy of the inhibitor, since the transition of an electron from HOMO to LUMO leads to a strong polarization of the double bond, a change in its order and, as a consequence, the formation of a covalent bond with the selenide ion Sec-498, the rate of this process determines the effectiveness of inhibition. Thus, our QSAR model representing inhibitor efficiency in the form of 1/lnIC50 values takes into account the contribution of the following molecular descriptors: Muliken charge, the electrostatic potential difference on beta and alpha atoms, Ovality, Electrostatic potential and HOMO energy [22, 23].
Table 2 presents the results of binding energy for covalent docking with a molecular descriptor.
The regression of the predicted half-inhibition concentration values with the experimental values and the pharmacophore model are presented in Fig. (1).
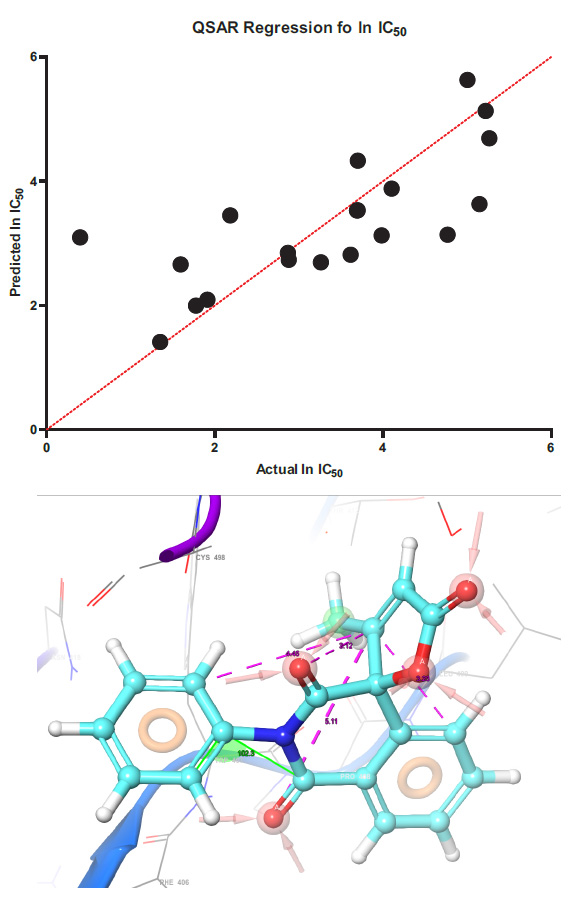
Y(lnIC50) = -6.795*B -23.83*C + 3.726*D -7.592*E -0.3010*F + 14.61*C2 + 41.65*E2
Regression models for determining the lnIC50 value using molecular descriptors. Mathematical model of regression equation Where: B – Mulliken charge, C – ovality, D – charge difference on the alpha and beta atoms of butenolide, E – HOMO energy F Electrostatic potential of the beta atom. Statistically, data we determine in GraphPad prism in PLS algorithm in absolutely values model confidence interval have ± 12,58 mkmol.
Entry | IC 50 μM | ln IC 50 μM | Mulliken Charge | Ovality | Δq α and β | Electrostatic β | E HOMO | ΔG Covalent Docking kcal/mol |
---|---|---|---|---|---|---|---|---|
1 | 1.49 | 0.398776 | -0.029 | 1.42 | 0.095 | -0.185 | -6.63 | -47.89 |
2 | 40.00 | 3.688879 | -0.041 | 1.45 | 0.140 | -0.145 | -6.40 | -44.09 |
3 | 8.87 | 2.182675 | 0.097 | 1.51 | 0.000 | -0.200 | -6.78 | -45.62 |
4 | 117.60 | 5.145749 | -0.025 | 1.49 | 0.048 | -0.201 | -6.09 | -54.88 |
5 | 17.78 | 4.767289 | 0.035 | 1.40 | 0.149 | -0.211 | -6.39 | -54.88 |
6 | 54.20 | 2.878074 | -0.024 | 1.41 | 0.056 | -0.145 | -6.74 | -42.88 |
CONCLUSION
As a result of modeling the binding process of electron-deficient olefins in the active center of thioredoxin reductase with a selenocysteine residue, we found that the rate of the binding process is limited by the formation of a complex between the inhibitor and the enzyme. Interaction with residues Lys-29, Thr-481 and Gln – 494 ensures the necessary conformational changes of the inhibitor in the active site, which allows obtaining the necessary gain in energy to provide the binding of the inhibitor. The presence of a methoxy substituent in the aryl fragment near the reaction center is preferred. This fact is associated with ensuring optimal polarization of the double bond of the electron-deficient olefin. The sites of contact with the residues of the side groups of the active center must be aromatic and hydrophobic, at the same time, the conformational mobility of the double bond of the electron-deficient olefin provides sufficient freedom for successful interaction with the selenium atom. The presence of donor substituents at the nitrogen atom of the homophthalemide fragment leads to a decrease in the electrostatic charge on the beta atom of furanone, which reduces its electrophilicity and, as a consequence, the rate of reaction with the selenium atom SEC 498. Alkyl substituents are less preferred in this case. In general, QSAR analysis allows us to present a pharmacophore model for unsaturated spiranolactones and furanones. At the moment, our group has obtained an extensive library of new spirobutenolides, as well as new electron-deficient olefins based on benzylidene succinimides, we plan for 120 new compounds to calculate molecular descriptors and semi-inhibition concentration values using our model, perform a colorimetric determination of inhibition activity in a wet experiment and test the stability and the accuracy of the model, which we hope to publish in the next article. This publication opens a series of works on the rational design of thioredoxin reductase inhibitors using methods QSAR.
AUTHOR’S CONTRIBUTION
It is hereby acknowledged that all authors have accepted responsibility for the manuscript's content and consented to its submission. They have meticulously reviewed all results and unanimously approved the final version of the manuscript.
LIST OF ABBREVIATIONS
Trx R1 | = Thioredoxin reductase I |
PDB | = Protein Data Bank |
SP | = Standard Precision |
DFT | = Density Functional Algorithm |
PBS | = Phosphate Buffer Solution |
AVAILABILITY OF DATA AND MATERIALS
The data and supportive information are available within the article.