All published articles of this journal are available on ScienceDirect.
Computational Design of Multitargeted Ligands to Counter Drug Resistance of Mycobacterium Tuberculosis
Abstract
Background
Tuberculosis (TB) is a significant global health challenge due to drug resistance. Furthermore, tuberculous meningitis (TBM), which affects the central nervous system, has a particularly high mortality rate. TBM drugs have low efficacy because of their low blood-brain barrier (BBB) permeability. Many institutions that treat tuberculosis lack the infrastructure to identify specific drug-resistance mutations. The development of drugs with the capability of treating multiple strains would contribute considerably to the advancement of TB control in countries with limited resources. Therefore, there is an urgent requirement for novel therapeutics that can target native and drug-resistant strains.
Objective
This study aimed to design a novel drug to target native as well as drug-resistant Mycobacterium tuberculosis (MTB) strains associated with pulmonary TB and TBM.
Methods
RNA Polymerase beta-subunit (rpoB) was chosen because it is a validated target for MTB. Pharmacophore features, core moiety analysis, and docking scores were used for ligand screening. Deep neural networks (DeepFrag) were used for structural optimization, and binding affinity was evaluated using AutoDock Vina. Custom scoring schemes, STWMM for TB and STWMMM for TBM, met the requirements of high binding affinity for multiple targets, optimal pharmacokinetic profiles, and chemical synthesizability.
Results
M1, M2, and M3 were the molecules with the highest STWMM and STWMMM scores, indicating their potential for TB and TBM therapy. The average binding energy of M1 was -8.83 kcal/mol for native and mutant rpoB. The average binding energy for M2 and M3 was -9.63 and -9.83 kcal/mol, respectively).
Conclusion
In this study, novel ligands for native and drug-resistant TB and TBM therapy were obtained by multi-target drug design. A major challenge for current therapeutic regimens for TB and TBM is the rise of drug-resistant strains of Mycobacterium tuberculosis and the necessity of distinguishing them from the native strains. The multitargeted ligands developed in this study have the potential to overcome these limitations.
1. INTRODUCTION
Tuberculosis (TB) is one of the deadliest infectious diseases, competing with AIDS and malaria [1, 2]. Despite being a preventable and curable illness, tuberculosis remains a primary cause of global mortality and morbidity. In 2022, tuberculosis was the second leading cause of death from a single infectious pathogen, surpassed only by COVID-19. It claimed about twice as many lives as HIV/AIDS, demonstrating its public health impact [3]. RNA polymerase beta-subunit (rpoB) plays a critical role in transcription and is essential for the survival of Mycobacterium tuberculosis. Its extremely conserved structure and its role in essential bacterial functions further support the selection of rpoB as an attractive and critical target for therapeutic intervention [4-6]. RpoB is the target of rifampicin, one of the most efficient first-line anti-TB agents. The mutations occurring in this well-characterized gene are by far the most significant cause of rifampicin resistance and are a major barrier to therapy in multidrug-resistant (MDR) and extensively drug-resistant (XDR) cases. The design strategy presented in this work aims to overcome the challenge of drug resistance across a broad spectrum of MTB strains, including those involved in tuberculous meningitis (TBM), by designing inhibitors that bind effectively to native and mutant rpoBs. This multi-target approach has the potential to overcome the limitations of the current TB and TBM therapies.
Tuberculous meningitis (TBM), a severe manifestation that affects the central nervous system, is associated with high rates of morbidity and mortality [7, 8]. It is caused by Mycobacterium tuberculosis and can result in a variety of neurological problems if not treated on time [9, 10]. TBM mortality rates can exceed 50%, especially in cases in which the illness is not detected early [11]. This burden worsens in individuals who are co-infected with HIV and have drug-resistant strains of tuberculosis [12, 13]. TBM is a complicated disease that poses a significant challenge to global health. Therefore, improving treatment outcomes requires an understanding of the underlying mechanisms of TBM, particularly those linked to drug resistance [14-16].
Tuberculous meningitis causes clinical problems with a wide range of symptoms, such as fever, headache, altered mental status, and neurological abnormalities [10]. The acid-fast bacilli (AFB) smear test is one example of a traditional diagnostic technique that has low sensitivity, especially in countries with limited resources where it is most frequently used [17, 18]. More sensitive diagnostic methods, such as the GeneXpert MTB/RIF, have been widely adopted. However, availability is still restricted in many high-burden areas, resulting in a large number of untreated or unconfirmed cases [19-21].
The current first-line therapy for tuberculosis, recommended by WHO, includes rifampicin, isoniazid, pyrazinamide, and ethambutol [22]. Although this therapeutic regimen is effective for pulmonary TB, its effectiveness is substantially reduced for MDR-TB [23].
Rifampicin (RMP) is a key component of TB treatment, targeting the RNA polymerase enzyme, specifically the beta-subunit encoded by the rpoB gene [24, 25]. Concerning patterns in medication resistance to tuberculosis have been reported by recent epidemiological research, especially in rifampicin-resistant strains of the disease that include mutations in the RNA polymerase beta-subunit [26-28]. Comprehending the resistance mechanisms facilitated by these mutations is crucial in order to develop focused therapies that enhance the management of TBM [29].
It has been reported that the most common mutations (65-86%) occur at positions 451 and 456 of rpoB. The emergence of RIF-resistant strains, harboring mutations, such as S456L, H451D, H451P, H451Y, and D441V within the RNA polymerase beta-subunit, poses a significant obstacle to effective TB treatment [30-32]. The effectiveness of the drug is decreased by these mutations because they alter the RIF binding site on RNA polymerase. Treatment becomes even more challenging due to M. tuberculosis's tendency to develop fitness-compensatory mutations [33]. By enabling the resistant strains to survive and replicate even in the absence of the medication, these changes can lessen the fitness costs associated with drug resistance, extending the duration of resistant bacterial populations in the host and the community [34, 35]. Developing innovative therapies against tuberculosis (TB) by targeting the RNA polymerase beta-subunit is a potential path, particularly when considering drug-resistant strains [36, 37].
RpoB mutations are present in 96.1% of RIF-resistant MTB strains globally [38-40]. Most of the drug resistance strains have mutations in the Rifampicin Resistance Determining Region (RRDR), which is found close to the active site amino acid residues 432-458 (amino acids 507–533 according to E.coli numbering) of RNA polymerase beta-subunit. These mutations are also found in TBM, which affects the efficacy of treatment methods [41, 42]. A study in India found the presence of drug-resistant mutations in TBM patients, highlighting the challenge of managing drug-resistant TBM [43]. A study in South Africa revealed a high frequency of multi-drug-resistant (MDR) TB strains in TB cases, with rpoB mutations at positions 451 and 456 playing a key role [44].
Resistance mutations are not only confined to rpoB but also include changes in other genes, such as katG and inhA, which are implicated in isoniazid activation and function [45-48]. The prevalence of resistant mutants differs by geographic region and population [49, 50]. Isoniazid activation requires the katG gene, which encodes catalase-peroxidase. Mutations in katG can cause isoniazid resistance. According to reports, katG mutations are common in multidrug-resistant (MDR) TB strains and occur in a large proportion of TB patients [51]. Approximately 30% of MDR-TB cases have katG mutations, which complicate treatment regimens. Mutations in inhA lead to isoniazid resistance and are present in around 15-20% of resistant TB strains.
Besides rpoB, katG, and inhA, mutations in genes like embB and pncA have been related to resistance to ethambutol and pyrazinamide, respectively [52-54]. Although less common, these mutations add to the overall complexity of TB treatment.
Drugs, such as bedaquiline, pretomanid, linezolid, and moxifloxacin, which are novel drugs with different targets, have been shown to be more effective than the current first-line therapeutic regimen for MDR-TB [55]. However, since drug resistance may develop for these novel drugs also, the usage of the new drugs is only recommended for cases where resistance to the first-line therapeutic regimen is confirmed [56]. Identification of drug resistance based on lack of response may lead to undesirable irreversible consequences in some cases, and many institutions that are responsible for the treatment of TB do not have the facilities to determine the drug resistance profile. The goal of this work is to design multitargeted drugs that are effective against native rpoB as well as the common mutants that confer drug resistance to Mycobacterium tuberculosis so that they can be prescribed as a replacement for rifampicin without knowledge of the state of drug resistance.
The Mycobacterium tuberculosis (MTB) rpoB mutants (S456L, H451D, H451P, H451Y, and D441V) were chosen for this study based on their reported role in mediating drug resistance. Numerous reports have linked these changes to resistance to rifampicin, one of the major antibiotics used in combating tuberculosis (TB). Although these changes are frequently seen in pulmonary tuberculosis (TB), there is growing evidence that drug-resistant strains are also associated with tuberculous meningitis (TBM) [11, 57, 58].
Research indicates that TBM cases reflect the same drug-resistance mechanisms as pulmonary tuberculosis, with rpoB gene mutations being a major factor in reducing the efficacy of conventional therapies [59, 60]. This is a serious problem for the management of TBM since drug resistance can seriously impair the effectiveness of current treatment methods [61]. It is important to address these rpoB mutations in order to develop novel therapeutic approaches, particularly for drug-resistant cases of TBM when traditional therapies may not be effective.
In this study, in silico approaches were utilized to design novel therapeutic agents to target rpoB and its mutants. Deep neural networks, molecular docking, and advanced scoring schemes were used to design, screen, and rank potential drug candidates based on their predicted binding affinity and efficacy against TB and TBM. The objective of this study was to design multitargeted ligands for effective treatment of drug-susceptible and drug-resistant M. tuberculosis strains to improve the treatment outcomes for patients with pulmonary TB as well as TBM.
2. METHODOLOGY
2.1. Target Preparation
Although seventy-one structures of rpoB of Mycobacterium tuberculosis are available in the RCSB PDB, these experimentally obtained structures are either of low resolution that is not adequate for accurate computational docking studies or structural information regarding some fragments is missing. Therefore, the structure of the native molecular target, rpoB, was obtained from the AlphaFold server known for its high-accuracy predictions of protein structures from amino acid sequences [62]. This structure was used as the reference for our docking studies. For the drug-resistant mutants of rpoB, including S456L, H451D, H451P, H451Y, and D441V, the mutant models were generated using the Robetta server [63], which is capable of generating 3D protein structures with high accuracy. AutoDock Tools (ADT), an extensively used and validated software package for preparing docking simulations, was utilized to process the receptor structures, which involved the removal of water molecules, the addition of polar hydrogens, and the selection of rotatable bonds. All side chains in the receptor structure were defined as non-rotatable.
2.2. Ligand Preparation
2.2.1. Initial Screening
A set of 2041 natural product molecules (sourced from www.selleckchem.com) were screened for binding affinity against the rpoB S456L mutant protein using AutoDock4 [64]. For each molecule, AutoDockTools were used to prepare it as a 3D structure with the required atom types, charge assignments, and hydrogen addition. Then, all these prepared ligands were subjected to docking using AutoDock 4. Each ligand was docked into the active sites of mutant rpoB protein, and the docking pose binding energy for each ligand was recorded.
2.2.2. Identification of Binding Site Pockets
AutoDock Tools were used to define the binding site pockets of rpoB S456L. The grid box was centered over a predicted site of activity on the rpoB S456L mutant, which was identified in earlier studies on the native RNA polymerase beta subunit. The grid was selected to be large enough to include the main catalytic site but also the secondary binding pockets. A 0.375 Å spacing in the grid was selected, meeting demands for precision with computational efficiency. This box served as the docking area for the virtual screening, ensuring that only relevant conformations of the ligands were considered for further analysis.
2.2.3. Screening Process
Each of the 2041 natural product molecules was subjected to virtual screening within the defined site pockets. The Lamarckian genetic algorithm was applied for the docking simulations. This algorithm explores ligand conformations within the binding site, optimizing the ligand’s orientation and conformation to find the lowest-energy binding mode. Each molecule was docked multiple times to ensure the consistency of results. After docking, ligands were ranked based on their binding energy and their interactions with key residues in the pocket. The top-scoring molecules were selected for further analysis.
Following screening, the core moiety structures of the top-docked molecules were analyzed by using Avogadro [65], a molecular editing and visualization software. It was used to examine the three-dimensional structures, analyze molecular interactions, and identify key functional groups that could influence binding affinity and pharmacokinetic properties. This structural analysis led to the design of 32 novel molecules. The ADME (Absorption, Distribution, Metabolism, and Excretion) properties of these molecules were assessed by using SWISS-ADME [66]. The BBB permeability of the molecules was assessed using the PKCSM (https://biosig.lab.uq.edu.au/pkcsm/) [67] tool to determine their ability to cross the blood-brain barrier, which is crucial for potential central nervous system (CNS) therapeutics.
2.2.4. Advanced Docking
From the initial screening, 32 molecules were chosen based on their binding energies and interactions with the rpoB S456L mutant. These molecules were docked into the active site of the rpoB S456L mutant protein using AutoDock Vina [68], which was employed for precise binding energy calculations. A grid box for docking was prepared with the active site at the center, with dimensions of 66 x 74 x 72 Å and grid spacing of 0.375 Å by using AutoDock Tools. The docking output, consisting of 10 conformers and their affinity scores, was visualized by using Pymol. These 10 molecules were further docked with rpoB and the following drug-resistant mutants of rpoB: D441V, H451D, H451P, and H451Y.
2.2.5. Optimization with DeepFrag
To further enhance the binding affinity of the identified molecules, the top 10 molecules were subjected to optimization using DeepFrag (https://durrantlab.pitt.edu/deepfrag/) [69], a deep learning-based fragment optimization tool. This optimization process involved generating and evaluating novel molecular fragments to improve binding scores and refine interactions with the target proteins. This integrated approach facilitated the design and optimization of 100 novel molecules, combining computational screening, detailed docking with binding energy calculations, and advanced fragment optimization to identify and refine potential therapeutics for targeting rpoB mutants. From the 100 molecules designed and optimized through this integrated approach, the top 10 molecules were selected based on their superior binding affinity, chemical synthesizability, favorable ADME properties, and effective BBB permeability.
2.3. Scoring Scheme
The AutoDock Vina scoring function was employed to evaluate the binding affinity of each ligand. Vina’s scoring function estimates the free energy of binding based on ligand-receptor interactions, including van der Waals forces, electrostatic interactions, hydrogen bonding, and torsional flexibility. In addition to binding affinity, the pharmacokinetic attributes and chemical synthesizability were included in the scoring function. Lipinski, Ghose, Veber, Egan, and Muegge are logical values that are non-zero for drug-like compounds and were estimated by using the SWISS-ADME server. Lead-likeness is a logical value and is non-zero for lead like ligands estimated by using the SWISS-ADME server. Synthetic accessibility scores (SA) [70] can be calculated from fragment contributions and complexity analysis. In this study, they were obtained using the SWISS-ADME server. SA values range from 1 for easily synthesizable ligands to 10 for ligands that are very difficult to synthesize. logBB_pkCSM is a quantitative estimate of BBB permeability obtained by using the pkCSM server. BBBpermeability_F is a logical value that is non-zero for ligands predicted to be BBB permeable using the SWISS-ADME server.
2.3.1. STWMM Score
A weighted score, STW score, was calculated to include terms for pharmacokinetics and chemical synthesizability in addition to the AutoDock Vina score. It was calculated using the following formula:
STWscore = -0.5 * Vina Score + 1.0 * Lipinski_F + 0.125 * Ghose_F + 0.125 * Veber_F + 0.125 * Egan_F + 0.125 * Muegge_F + 1.0 * Bioavailability + 0.5 * Lead-likeness_F + 2.0 * (10 – SA) / 9
The last term of the STWscore was designed to have a maximum value of 2.0 for SA=1 and a minimum value of 0 for SA=10. The weights for the STWscore were selected to balance the requirements of affinity (pharmacodynamics), pharmacokinetics, and synthetic accessibility. STWscore is not a normalized score, and there is no maximum or minimum. For an excellent ligand with an AutoDock Vina score of -10.0 kcal/mol, bioavailability of 1.0, and synthetic accessibility of 1, which satisfies all the rules for drug-like molecules, the STWscore will be 10.0, with a 50% score due to binding affinity, 20% from synthetic accessibility, and 30% from other pharmacokinetic properties. Higher scores are possible for molecules with higher affinity.
The STWscore was calculated for each ligand of interest for the native form of the target as well as for each of the mutants. Furthermore, the STWMM score for the ligand was calculated as the minimum of the STWscores for the native and mutants that were under consideration, as follows:
STWMM Score = MIN {Native_STWscore, S456L_STWscore, H451D_STWscore, H451P_STWscore, H451Y_STWscore, D441V_STWscore}
2.3.2. STWMMM Score
A weighted score, STWNscore, was calculated to include terms for BBB permeability, pharmacokinetics, and chemical synthesizability, in addition to the AutoDock Vina score [71].
STWNscore = -0.5 * Vina Score + logBB_pkCSM + 0.25 * BBBpermeability_F + 1.0 * Lipinski_F + 0.125 * Ghose_F + 0.125 * Muegge_F + 0.5 * Lead-likeness_F + 2.0 * (10 – SA) / 9
The last term of STWN score was designed to have a maximum value of 2.0 for SA=1 and a minimum value of 0 for SA=10. The weights for the STWNscore were selected to balance the requirements of affinity (pharmacodynamics), permeability through the BBB, pharmacokinetics, and synthetic accessibility. STWNscore is not a normalized score, and there is no maximum or minimum. For an excellent ligand with an AutoDock Vina score of -10.0 kcal/mol, a logBB of 1.0, and synthetic accessibility of 1, which is predicted to be BBB permeable and satisfies all the rules for drug-like molecules, the STWNscore will be 10.0, with 50% of score due to binding affinity, 20% from synthetic accessibility, 12.5% contribution from BBB permeability, and 17.5% from other pharmacokinetic properties. Higher scores are possible for molecules with higher affinity or higher logBB values.
The STWNscore was calculated for each ligand of interest for the native form of the target as well as for each of the mutants. The STWMMM score for the ligand was then calculated as the minimum of the STWNscores for the native and mutants that were under consideration, as follows:
STWMMM score = MIN {Native_STWNscore, S456L_STWNscore, H451D_STWNscore, H451P_STWNscore, H451Y_STWNscore, D441V_STWNscore}
3. RESULTS
AutoDock Vina is a widely used open-source software for molecular docking, developed to predict the binding mode and affinity of small molecules to their target proteins. AutoDock Vina uses a scoring function, which, in turn, depends on various parameters like van der Waals interactions, electrostatic interactions, hydrogen bonding, desolvation effects, etc., to estimate the free energy of binding, i.e., docking energy, which is a crucial parameter in evaluating the potential of a molecule as a drug candidate. This energy is expressed in kilocalories per mole (kcal/mol). A more negative value indicates a stronger and more favorable interaction between the ligand and the target. Free energy of binding of -8.5 kcal or better (more negative) indicates a high affinity of the ligand for the protein [75-77].
The blood-brain barrier (BBB) is a selective, semi-permeable barrier that separates the circulating blood from the brain's extracellular fluid in the central nervous system (CNS). It serves as a critical checkpoint, protecting the brain from potentially harmful substances while allowing essential nutrients to pass through it [78]. For drugs intended to treat neurological conditions, the ability to cross the BBB is a vital consideration in drug design. LogBB is the log of the ratio of the concentration of the ligand in the brain to its concentration in the blood. Ligands with logBB > 0.3 are generally able to cross the blood-brain barrier, while molecules with logBB < -1 have poor access to the brain [67]. All the designed ligands, reported in Table 1, have a logBB value greater than 0.3.
S.No. | Molecule Name | Structure | STWMM Score | STWMMM Score | Swiss-ADME Synthetic Accessibility |
---|---|---|---|---|---|
1 | M1 |
![]() |
7.51 | 7.37 | 4.04 |
2 | M2 |
![]() |
7.48 | 7.56 | 3.8 |
3 | M3 |
![]() |
7.45 | 7.38 | 4.82 |
4 | M4 |
![]() |
7.30 | 7.15 | 4.89 |
5 | M5 |
![]() |
7.24 | 7 | 5.25 |
6 | M6 |
![]() |
7.21 | 7.01 | 4.89 |
7 | M7 |
![]() |
7.15 | 7.02 | 5.89 |
8 | M8 |
![]() |
6.91 | 6.75 | 5.7 |
9 | M9 |
![]() |
6.84 | 6.78 | 4.47 |
10 | M10 |
![]() |
6.69 | 6.5 | 4.2 |
The evaluation of novel therapeutic molecules targeting RNA polymerase mutants was conducted using two distinct scoring schemes: the STWMMM score, which focuses on drug-resistant mutations (DRMs) relevant to tuberculous meningitis, and the STWMM score, which encompasses DRMs in Mycobacterium tuberculosis (general). The top ten molecules based on their STWMM score are presented in Table 1. The AutoDock Vina scores of designed ligands for the native and rpoB mutant targets are presented in Table 2.
The binding energies reflect the interactions between the ligands and the target proteins, which are crucial for evaluating the therapeutic potential of these compounds against native and drug-resistant Mycobacterium tuberculosis. The data reported in Table 2 show that the designed molecules, M1-M7, have a binding energy of -8.5 kcal/mol or better for native as well as for all the mutants of rpoB that were included in this study. M8 has a binding energy of -8.5 kcal/mol or better for native and for all the mutants that were included in this study, except against the mutant target H451Y (-8.05 kcal/mol). M9 and M10 have a binding energy of -8.5 kcal/mol or better for native and for all the mutants that were included in this study, except against the mutant targets H451Y and H451P.
Table 1 presents the STWMM scores, STWMMM scores, and synthetic accessibility for the top ten designed ligands targeting the native RNA polymerase beta subunit and mutants observed in drug-resistant strains of Mycobacteriumtuberculosis. As mentioned in Table 1, the top three molecules are M1, M2, and M3 based on their STWMM scores.
Molecule M1, with the highest STWMM score of 7.51, shows an average AutoDock Vina score of -8.83 kcal/mol. This suggests a favorable binding affinity across different mutants, indicating its potential as a strong candidate for further development. Specifically, its binding energies range from -8.52 kcal/mol to -9.51 kcal/mol, demonstrating consistency in binding across the S456L, H451D, H451P,
S.No. | Molecule Name | AutoDock Vina Score (kcal/mol) | Average AutoDock Vina Score (kcal/mol) | |||||
---|---|---|---|---|---|---|---|---|
Rpob2 | S456L | H451D | H451P | H451Y | D441V | |||
1 | M1 | -8.84 | -9.51 | -8.63 | -8.87 | -8.59 | -8.52 | -8.83 |
2 | M2 | -9.87 | -10.92 | -9.02 | -9.14 | -8.6 | -10.24 | -9.63 |
3 | M3 | -10.4 | -10.44 | -10.31 | -9.33 | -9.0 | -9.49 | -9.83 |
4 | M4 | -10.37 | -10.67 | -10.05 | -9.52 | -8.73 | -9.02 | -9.73 |
5 | M5 | -9.75 | -10.59 | -9.95 | -9.82 | -8.52 | -8.93 | -9.59 |
6 | M6 | -9.81 | -11.25 | -9.19 | -8.55 | -8.94 | -8.93 | -9.45 |
7 | M7 | -10.18 | -10.05 | -9.55 | -9.29 | -8.63 | -9.44 | -9.52 |
8 | M8 | -9.15 | -10.1 | -10.02 | -9.65 | -8.05 | -9.98 | -9.49 |
9 | M9 | -9.59 | -9.25 | -8.68 | -7.62 | -8.47 | -8.77 | -8.73 |
10 | M10 | -9.17 | -9.95 | -8.86 | -7.21 | -8.12 | -8.86 | -8.70 |
H451Y, and D441V mutants. The high STWMM score, high STWMMM score, and the high binding affinity of M1 position it as a lead compound in the pursuit of new therapeutics for pulmonary tuberculosis as well as for tuberculous meningitis.
The interactions of molecule M1 with the active site of rpoB are illustrated in Fig. (1). M1 forms a hydrogen bond with Q614, and this interaction is critical for stabilizing the ligand within the binding pocket. Thus, this bond makes a significant contribution to the binding affinity of this ligand. There are two additional hydrogen bonds in this complex. One involves R613, which forms a medium-strength hydrogen bond, and another involves R454, which forms a weaker hydrogen bond. In addition to the formation of the hydrogen bond, R613 also forms a salt bridge to a carboxylate group in the ligand. In addition to these interactions, R613 stabilizes this complex through π-cation interactions. Hydrophobic interactions with V176, Q435, L436, L458, P489, and Q614 further stabilize the overall ligand-receptor complex, especially within the hydrophobic pocket of rpoB. These interactions firmly anchor the M1 molecule within the active site. The combination of hydrogen bonding, electrostatic, and hydrophobic interactions indicates that there is a strong possibility that M1 may retain tight binding against a variety of mutations, which is a prime concern in dealing with drug-resistant variants.
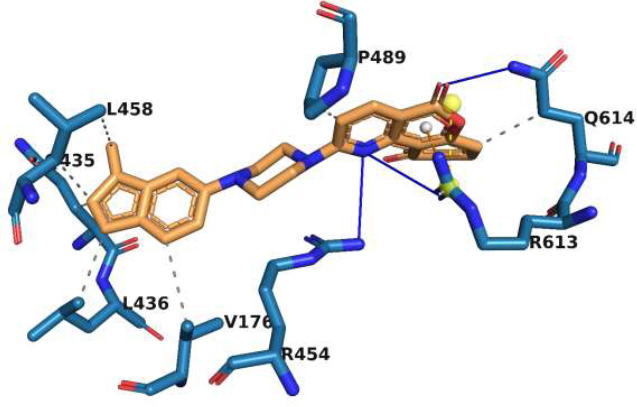
Interaction of molecule M1 with the active site residues of rpoB protein. The blue lines represent hydrogen bonds, the yellow dashed lines represent salt bridges, the orange dashed lines represent π-cation interactions, and the grey dashed lines represent hydrophobic interactions.
Molecule M2 exhibits an average binding energy of -9.63 kcal/mol, indicating it has one of the highest affinity among the designed compounds. Its individual binding scores, ranging from -8.60 kcal/mol to -10.92 kcal/mol, indicate that M2 has a high binding affinity for native rpoB as well as for all the mutants that were included in this study. The exceptional binding affinity of M2 indicates its potential as a therapeutic agent for TB and TBM. The slightly lower STWMM score of M2, compared to M1, indicates that M1 has better pharmacokinetic properties. However, the STWMMM score of M2 is higher than that of M1, indicating that it has better BBB permeability than M1, and hence, it may be more effective for TBM therapy compared to M1. It can be concluded that M2 will be effective for both TB and TBM.
The interactions of molecule M2 within the rpoB active site are shown in Fig. (2). Q435 forms two strong hydrogen bonds with M2. These interactions play a significant role in the stabilization of the rpoB-M2 complex. Π-cation interactions of the ligand with R454 further stabilize this complex. The ligand is anchored in the hydrophobic segment of the active site through its hydrophobic interactions with V176 and D441. In addition, weak van der Waal’s interactions with numerous residues in the active site stabilize this complex. The stabilization of this complex by numerous interactions indicates that the binding of M2 would be robust with respect to minor changes caused by point mutations in the target.
Molecule M3 has the best average AutoDock Vina score of -9.83 kcal/mol. The binding energies of M3, ranging from -9 kcal/mol to -10.4 kcal/mol, predict that it has a high binding affinity for native rpoB as well as for all the mutants that were included in this study, indicating that it is a potential candidate for drug development. Its consistent performance across the mutants reinforces its potential role in addressing resistance in combating drug-resistant Mycobacterium tuberculosis. Molecule M3 is ranked third for TB and second for TBM. The STWMM and STWMMM scores for M3 are lower than those for M1 and M2 because they have higher synthetic accessibility scores.
The binding interactions of molecule M3 to the active site of rpoB are illustrated in Fig. (3). M3 forms two strong hydrogen bonds with the backbone of F439, which is important because the backbone interactions are less prone to disruption from point mutations than interactions involving side chains. Binding of this nature by the backbone enables multitargeting, and it indicates the possibility that M3 could be active against a large number of rpoB mutants, including the most common resistance-associated mutations. M3 forms another strong hydrogen bond with R454. A salt bridge between D441 and a tertiary amine group in the ligand further stabilizes this complex. A strong hydrophobic interaction with F439 and additional hydrophobic interactions with Q438 and N679 firmly anchor M3 within the active site. The backbone hydrogen bond with F439 is a particularly strategic interaction for drug design, as it is less prone to alteration by mutations, which are a frequent cause of drug resistance. The diversity of non-covalent interactions, including van der Waals forces from multiple residues, increases binding affinity and makes this ligand resilient to structural variations induced by point mutation. Hence, M3 is a promising candidate for the treatment of drug-resistant strains of M. tuberculosis.
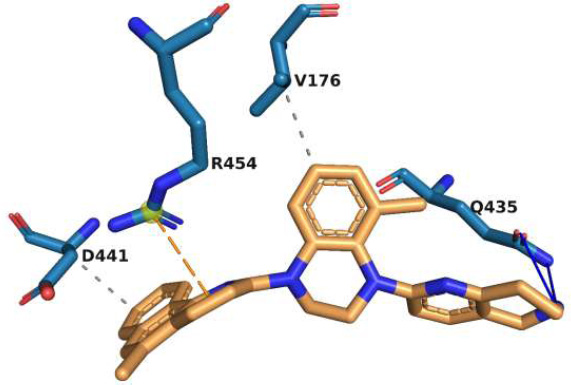
Interaction of molecule M2 with the active site residues of rpoB protein. The blue lines represent hydrogen bonds, the orange dashed lines represent π-cation interactions, and the grey dashed lines represent hydrophobic interactions.
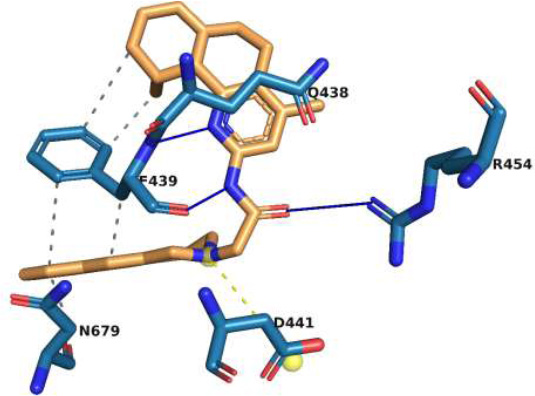
Interaction of molecule M3 with the active site residues of rpoB protein. The blue lines represent hydrogen bonds, the yellow dashed lines represent salt bridges, and the grey dashed lines represent hydrophobic interactions.
4. DISCUSSION
The first line of therapy, consisting of rifampicin, isoniazid, pyrazinamide, and ethambutol, recommended by WHO, is effective for the treatment of pulmonary tuberculosis [79]. However, this therapeutic protocol has a much lower efficacy for tuberculous meningitis because all components of this selected set have a low permeability through the blood-brain-barrier. Rifampicin has a pkCSM-logBB value of -2.1. The corresponding logBB values for isoniazid, pyrazinamide, and ethambutol are 0.00, -0.01, and -0.21, respectively, indicating that they are also expected to have low permeability through the BBB. Similarly, the new drugs developed for the treatment of pulmonary TB also have very low values of logBB. For example, bedaquiline, delamanid, and pretomanid have pkCSM-logBB values of 0.03, -2.1, and -1.7, respectively, indicating poor BBB permeability. All the designed ligands reported in Table 1 of this work have pkCSM-logBB values higher than 0.3, indicating adequate permeability through the BBB and the potential for higher efficacy of treatment for TBM. Customized drug delivery agents, such as nanoparticles, are being investigated to improve the permeability of rifampicin and isoniazid to improve the outcomes for tuberculous meningitis [80]. Drug resistance mutations have also decreased the efficacy of the first line of therapy. The BV-BRC database [81] includes descriptions of 25054 MTB strains resistant to rifampicin, 22022 MTB strains resistant to isoniazid, 2363 MTB strains resistant to pyrazinamide, and 9283 MTB strains resistant to ethambutol. Several novel drugs have been developed to overcome the drug resistance problem. However, these drugs are also susceptible to development of drug resistance. For example, 25 MTB strains resistant to bedaquiline and 571 MTB strains resistant to delamanid have been documented in the BV-BRC database. Although multiple-medication therapy, consisting of a combination of drugs, can be utilized, it can lead to undesirable adverse effects. The optimization of dosage and duration of each component is more complicated for drug combinations than for single drugs. The design of a single drug that has a high affinity for multiple targets provides an alternative solution that is potentially more efficient [82]. Multitargeted agents have been developed for the treatment of infectious diseases, such as malaria and Chagas disease [83, 84]. Designing poly-functional drugs is difficult using conventional methods. However, several computational strategies for the design of poly-functional drugs have been proposed, such as fragment-based design [85]. Our approach for multi-targeted drug design is also fragment-based, but the utilization of Deep Neural Networks has resulted in a substantial increase in the efficiency of the design process [69].
Rifampicin is one of the most effective therapeutics for pulmonary TB. However, the emergence of mutations in its target, the RNA polymerase beta subunit (rpoB), has greatly reduced its effectiveness. These mutations cause major changes to the rifampicin-binding site, lowering the drug's ability to suppress RNA synthesis, which is necessary for bacterial replication [86-88]. This study's major goal is to provide an effective therapeutic option for both pulmonary TB and tuberculous meningitis (TBM) by designing novel multitargeted ligands whose effectiveness would not be reduced by such mutations. In this study, we conducted an in silico design of novel ligands targeting RNA polymerase and its mutants.
TBM is a particularly severe form of tuberculosis involving infection of the central nervous system. TBM therapy requires the design of drugs that penetrate the blood-brain barrier (BBB), which is a very challenging problem [89, 90]. Current TB drugs, which have been developed primarily for pulmonary tuberculosis, frequently fail to permeate the BBB in sufficient concentrations to effectively combat TBM [91, 92]. This limitation emphasizes the importance of developing drugs that are not only effective against drug-resistant strains of M. tuberculosis but are also capable of crossing the BBB to treat central nervous system infections. This work is intended to generate such ligands by targeting rpoB and its mutations, with the goal of reducing the high mortality rate due to TBM.
RpoB was selected as the molecular target due to its involvement in bacterial transcription and because it has been validated as a target of Rifampicin, which is one of the most effective drugs against TB. Mutations in the Rifampicin Resistance-Determining Region (RRDR), including S456L, H451D, H451P, H451Y, and D441V, were chosen due to their high frequency in clinical isolates and their effect on rifampicin efficacy [93-95]. These mutations alter the rifampicin binding pocket of rpoB, lowering rifampicin binding affinity and, in many cases, resulting in a high level of drug resistance. The core moiety analysis, performed with Avogadro, was very important in refining the ligands' structural components. By identifying the most significant molecular fragments responsible for high-affinity binding, we were able to concentrate on ligands capable of overcoming the steric and electronic alterations caused by rpoB mutations. This level of structural insight is crucial. After preliminary screening, we used DeepFrag to design novel ligands. DeepFrag uses machine learning techniques to recommend changes to small compounds that improve their binding properties while preserving or improving their pharmacokinetic properties. This enabled a more in-depth exploration of the chemical structures, resulting in the discovery of molecules with increased binding potential for rpoB and its mutants.
Balancing the activity of the designed ligands, against the multiple targets of interest is critical to ensure that a high dose would not be required to ensure efficacy against all targets of interest [96]. The STWMM and STWMMM scoring schemes were developed to assess the ligands' potential efficacy against pulmonary tuberculosis and TBM, respectively. These scoring schemes include the most important criteria for effective drugs, integrating binding affinity, mutational resistance, pharmacokinetics, and chemical synthetic accessibility to provide a complete framework for evaluating the therapeutic potential of designed molecules. These scoring schemes were designed to address the problem that arises when the dominant variant of the pathogen is eliminated by the utilization of an antibiotic and the niche initially occupied by the drug-susceptible strain is rapidly occupied by a mutant that is initially present at low frequency in the initial population. The multi-targeted ligands selected by the STWMM and STWMMM scoring schemes ensure that the ligands with high STWMM and STWMMM scores have high efficacy against the native as well as against all the drug-resistant strains. In addition, since the designed multi-targeted ligands are effective against both the native and mutant strains, the importance of obtaining genotyping information, or for other tests of drug resistance, is less than that for conventional drugs.
This study goes beyond the computational aspects of drug design to highlight the broader consequences of targeting rpoB mutations. The prevalence of these mutations in clinical isolates of drug-resistant M. tuberculosis empFVhasizes the relevance of focusing on these targets in future treatment approaches. The S456L mutation, for example, has been shown to confer high-level resistance across a variety of geographic regions, making it an important target for therapeutic development. Similarly, changes like H451D and D441V complicate the treatment landscape since they not only provide resistance but also modify bacterial fitness, potentially allowing resistant strains to survive and spread.
Moreover, the challenges posed by TBM add an additional layer of complexity to TB treatment. TBM is associated with high mortality, particularly in regions with limited access to diagnostic tools and effective treatments. The ability of the designed ligands to cross the BBB and maintain efficacy in the central nervous system is a critical advancement. The study’s dual focus on both pulmonary TB and TBM reflects the real-world need for therapeutics that can address the diverse clinical manifestations of tuberculosis. Future experimental validation of these designed ligands is critical for ensuring their efficacy and safety. Expanding this approach to include other mutations and targets of other drugs could further enhance our ability to combat drug-resistant Mycobacterium tuberculosis.
CONCLUSION
In this study, we successfully designed novel multitargeted ligands as potential drugs for pulmonary TB and TBM. The designed ligands have a high affinity for both native as well as for mutant rpoB of Mycobacterium tuberculosis. Our approach involved molecular docking-based screening and ranking and deep neural network (DeepFrag) based optimization. We also examined how well these molecules might pass through the blood-brain barrier by calculating pkCSM BBB values, which is particularly important for the treatment of TBM, which affects the central nervous system. In addition, we assessed synthetic accessibility by using Swiss-ADME to ensure that these molecules could be produced efficiently. AutoDock Vina was employed to evaluate binding affinity for the native and mutant forms of rpoB (S456L, H451D, H451P, H451Y, and D441V), which are involved in resistance to current treatments for TB. Moreover, the designed molecules were assessed using novel scoring schemes, STWMM and STWMMM, that were designed for integrated assessment of the essential features desired in drugs, such as binding affinity, mutational resistance, pharmacokinetics, and synthesizability.
After a thorough analysis, three promising candidates, M1, M2, and M3, were identified. Each of these designed molecules was predicted to possess favorable pharmacokinetics, chemical synthesizability, and a high binding affinity towards native rpoB, as well as against all mutant variants that were included in this study. Based on these promising results, we conclude that the designed multitargeted molecules, M1, M2, and M3, offer a new treatment option for TB and TBM, especially in cases where drug resistance is a concern. Further in vitro and in vivo studies are warranted to confirm their effectiveness and potential for clinical use.
In conclusion, this study represents a significant step forward in addressing the urgent need for developing novel therapeutic approaches for TB and TBM by designing ligands with the capability to target both native and mutant strains of Mycobacterium tuberculosis.
AUTHORS’ CONTRIBUTION
V.V. and S.T.: Provided the conception and design of the study; V.V.: Collected the data, analyzed the data, and wrote the manuscript; S.T.: Assisted in target preparation, manuscript editing, and revision.
LIST OF ABBREVIATIONS
TB | = Tuberculosis |
TBM | = Tuberculous Meningitis |
CNS | = Central Nervous System |
HIV | = Human Immunodeficiency Virus |
rpoB | = RNA Polymerase beta-subunit |
RMP | = Rifampicin |
AFB | = Acid-Fast Bacilli |
RRDR | = Rifampicin Resistance Determining Region |
ADME | = Absorption, Distribution, Metabolism, and Excretion |
BBB | = Blood-Brain Barrier |
MTB | = Mycobacterium tuberculosis |
MDR | = Multidrug-Resistant |
AVAILABILITY OF DATA AND MATERIALS
All data generated or analysed during this study are included in this article.
ACKNOWLEDGEMENTS
Declared none.